The chatbot has made waves over the past couple of months for being able to answer queries in a conversational tone. CS professors discuss what it can and cannot do correctly.
OpenAI’s ChatGPT is an artificial intelligence (AI) chatbot that is trained to follow the instruction in a prompt and give a detailed response. It is built upon GPT-3, a type of large language model (LLM) that predicts and generates text. Given a sequence of words, it will predict the word that has the highest probability of following next (kind of like autocomplete). These models are trained on huge datasets that allow them to generate answers to questions. ChatGPT works quickly and gives answers within seconds, and it also learns from every interaction and improves daily.
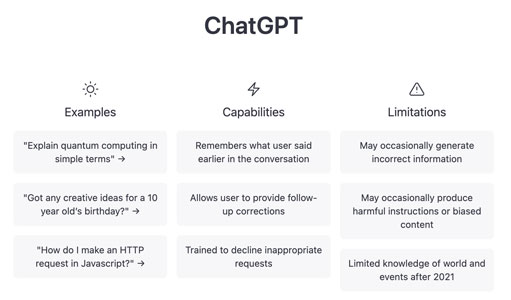
It can create a letter to your super asking for a repair to be done, write code and fix bugs, and suggest plot summaries for novels. But that does not mean that it is perfect. The problem with LLMs is that they can “hallucinate” and make things up. ChatGPT is guilty of this; some of the answers in its outputs do not even exist. It is also not trained to be truthful and it answers queries with a lot of confidence and authority, which is worrisome.
It is being compared to the last great tech disruption–the internet’s onset in the 1990s. We asked CS professors what the technology could do and how to use the tool the right way.
Vishal Misra
I have been using GPT-3 for over two years now. It is the underlying model behind my cricket search app for ESPN.
The original interface was cumbersome and needed an analyst who could use specialized programming languages to access the answer.
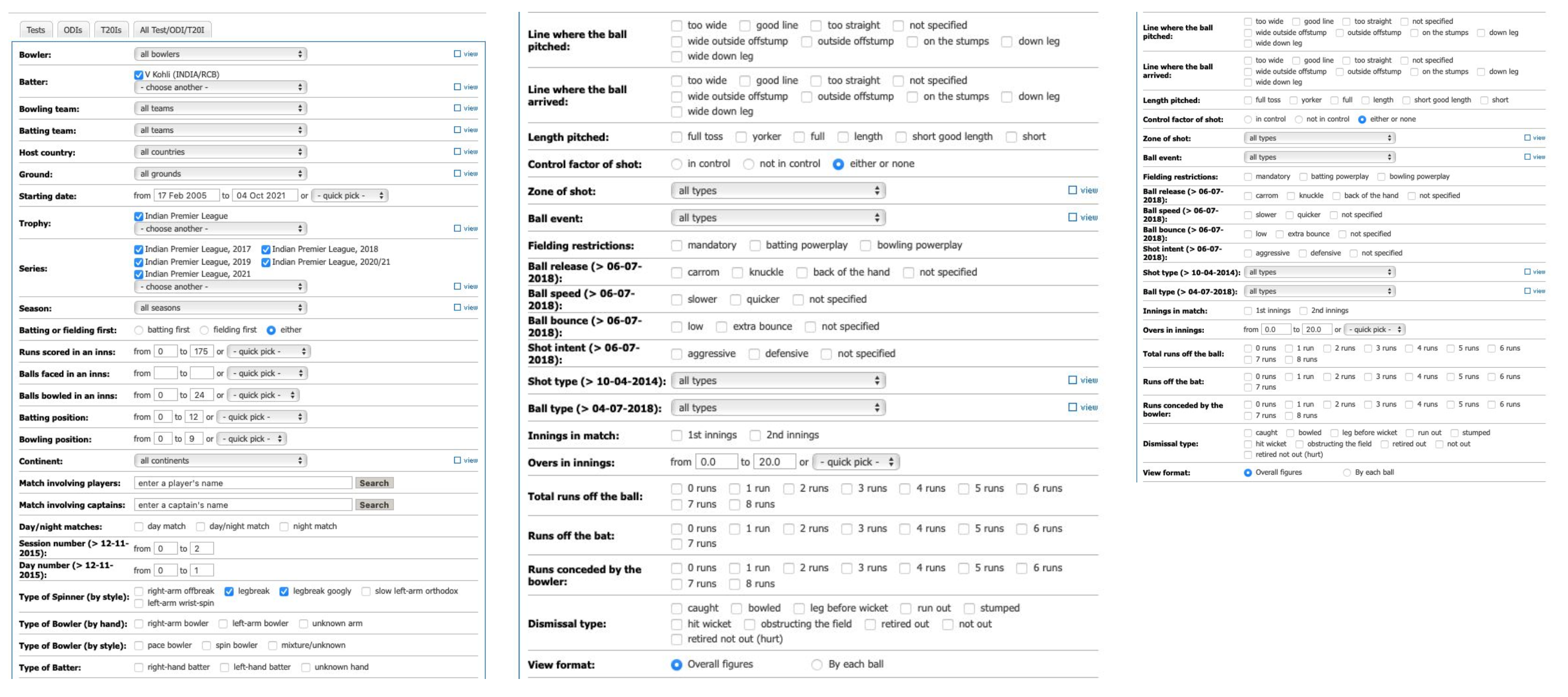
We developed AskCricInfo, which takes human input–questions or search queries–and converts the queries into a structured language like SQL that machines understand. The technology can “translate” the question into a programming language, find the answer, and quickly send it back to the user.
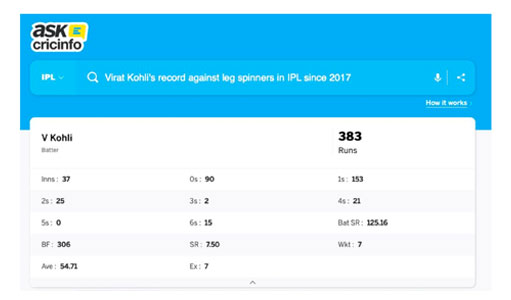
It is an excellent example of the power of underlying technology and what the tool can do. ChatGPT is very interesting. It is the first chatbot that makes “intelligent” and engaging conversations. There are definite use cases for making it a very effective teaching tool. It is up to the instructors to imagine a world where something like ChatGPT will always be available and teach students how to use it effectively. A lot can go wrong with ChatGPT- primarily because of its design that makes it bullshit confidently. But that is a problem with all LLMs, not just ChatGPT.
Kathy McKeown
ChatGPT and the technology it is built on, GPT3, are game changers. With them and the other large language models that have recently emerged, models can now produce extremely fluent text. They are also able to perform a variety of tasks in a way that was not previously possible. And there are many situations in which they can be used to help us do things that we previously found tedious. But they are not able to do as much as we might initially have imagined. They have limitations that are not always immediately obvious, and researchers are taking the time to study them so that we can accurately characterize what they can and cannot do. Nonetheless, they change how we do research in the natural language field and how we build new models to carry out other tasks.
I think we need to learn how to incorporate them into our teaching because they are here to stay. Students know about them and will know how to use them. We will need to think creatively about how to teach students what these tools do well and what they do poorly, when they can be used as a tool to aid in research, and situations in which it would be dishonest. I imagine situations where we ask ChatGPT to answer an essay question, ask the student to critique the essay and identify what it does poorly, and then ask the student to write an essay that goes beyond what ChatGPT can do.
The worst problem with ChatGPT is that it is not always truthful. In fact, it may often lie or hallucinate facts. It does this very fluently, making its lies sound very plausible. This is quite a barrier to use in a real-world situation unless it is used by someone who knows what is correct, what is not, and can sift the good from the bad. Technology constantly changes, though, and it is a problem that researchers are actively working on.
Itsik Pe’er
I asked ChatGPT about AI language models, and this is the output:
AI language models like ChatGPT, its competitors and successors represent a significant advancement in the field of Natural Language Processing (NLP). These models have the ability to generate human-like text based on a massive amount of input data, making them useful for various applications such as chatbots, question-answering systems, and content generation.
However, it is important to note that despite their sophisticated capabilities, they still have limitations and may generate outputs that are inaccurate, biased, or inappropriate. It is crucial to ensure responsible development and deployment of these models to maximize their benefits while minimizing potential harm.
The above demonstrates my thoughts more than I could otherwise express. In particular: it is already useful, but often not out-of-the-box, but I am a techno-optimist and believe even more usefulness is coming.
As a former judge in high school debate competitions, it reminds me of the most eloquent contestants, who would, if they needed to, lie through their teeth without missing a heartbeat. For instance, in response to this prompt, “What could go wrong with tools like ChatGPT?”, it writes:
There are several potential risks associated with the use of AI language models like ChatGPT:
Bias: AI models are trained on large amounts of text data, which may reflect societal biases and lead to biased outputs.
Misinformation: AI models may generate false or misleading information, which could have serious consequences if relied upon.
Privacy Concerns: AI models process large amounts of personal information, raising privacy and security concerns.
Misuse: AI models may be used for malicious purposes, such as spreading hate speech, fake news, or conducting phishing attacks.
Job Loss: AI models may automate certain tasks, leading to job loss in industries that rely on those tasks.
Therefore, it is important to carefully consider the potential risks and consequences of AI models and to develop and deploy them responsibly to minimize potential harm.
These are all valid points, but more context needs to be considered:
- When it comes to bias, chatbots are worse than the best other (human?) alternatives but much better than the median ones.
- Misinformation is a big problem when compounded by misuse, and we can see that happening now with stories posted online.
- Privacy concerns depend on who has access to the data in ChatGPT and what can be done with it. But given that we all carry powerful tracking and sensing devices 24/7, our privacy is already weakly protected against powerful actors.
- Some attention-seeking blogs and websites overstate the job loss point. Many jobs will become more efficient; many jobs will change; many jobs will be created, and, yes, many will be lost. People will adapt, and we will all be better for it.
Teaching AI to distinguish between causation and correlation would be a game changer— well, the game may be about to change.
The J.P. Morgan AI Research Awards 2021 partners with research thinkers across artificial intelligence.
Research from the department has been accepted to the 2021 Computer Vision and Pattern Recognition (CVPR) Conference. The annual event explores machine learning, artificial intelligence, and computer vision research and its applications.
Open-Vocabulary Object Detection Using Captions
Alireza Zareian Snap Inc. and Columbia University, Kevin Dela Rosa Snap Inc., Derek Hao Hu Snap Inc., Shih-Fu Chang Columbia University
Abstract
Despite the remarkable accuracy of deep neural networks in object detection, they are costly to train and scale due to supervision requirements. Particularly, learning more object categories typically requires proportionally more bounding box annotations. Weakly supervised and zero-shot learning techniques have been explored to scale object detectors to more categories with less supervision, but they have not been as successful and widely adopted as supervised models. In this paper, we put forth a novel formulation of the object detection problem, namely open-vocabulary object detection, which is more general, more practical, and more effective than weakly supervised and zero-shot approaches. We propose a new method to train object detectors using bounding box annotations for a limited set of object categories, as well as image-caption pairs that cover a larger variety of objects at a significantly lower cost. We show that the proposed method can detect and localize objects for which no bounding box annotation is provided during training, at a significantly higher accuracy than zero-shot approaches. Meanwhile, objects with bounding box annotation can be detected almost as accurately as supervised methods, which is significantly better than weakly supervised baselines. Accordingly, we establish a new state-of-the-art for scalable object detection.
Vx2Text: End-to-End Learning of Video-Based Text Generation From Multimodal Inputs
Xudong Lin Columbia University, Gedas Bertasius Facebook AI, Jue Wang Facebook AI, Shih-Fu Chang Columbia University, Devi Parikh Facebook AI and Georgia Tech, Lorenzo Torresani Facebook AI and Dartmouth
Abstract
We present Vx2Text, a framework for text generation from multimodal inputs consisting of video plus text, speech, or audio. In order to leverage transformer networks, which have been shown to be effective at modeling language, each modality is first converted into a set of language embeddings by a learnable tokenizer. This allows our approach to perform multimodal fusion in the language space, thus eliminating the need for ad-hoc cross-modal fusion modules. To address the non-differentiability of tokenization on continuous inputs (e.g., video or audio), we utilize a relaxation scheme that enables end-to-end training. Furthermore, unlike prior encoder-only models, our network includes an autoregressive decoder to generate open-ended text from the multimodal embeddings fused by the language encoder. This renders our approach fully generative and makes it directly applicable to different “video+x to text” problems without the need to design specialized network heads for each task. The proposed framework is not only conceptually simple but also remarkably effective: experiments demonstrate that our approach based on a single architecture outperforms the state-of-the-art on three video-based text-generation tasks—captioning, question answering, and audio-visual scene-aware dialog. Our code will be made publicly available.
Co-Grounding Networks With Semantic Attention for Referring Expression Comprehension in Videos
Sijie Song Wangxuan Institute of Computer Technology, Xudong Lin Columbia University, Jiaying Liu Wangxuan Institute of Computer Technology, Zongming Guo Wangxuan Institute of Computer Technology, Shih-Fu Chang Columbia University
Abstract
In this paper, we address the problem of referring expression comprehension in videos, which is challenging due to complex expression and scene dynamics. Unlike previous methods which solve the problem in multiple stages (i.e., tracking, proposal-based matching), we tackle the problem from a novel perspective, co-grounding, with an elegant one-stage framework. We enhance the single-frame grounding accuracy by semantic attention learning and improve the cross-frame grounding consistency with co-grounding feature learning. Semantic attention learning explicitly parses referring cues in different attributes to reduce the ambiguity in the complex expression. Co-grounding feature learning boosts visual feature representations by integrating temporal correlation to reduce the ambiguity caused by scene dynamics. Experiment results demonstrate the superiority of our framework on the video grounding datasets VID and OTB in generating accurate and stable results across frames. Our model is also applicable to referring expression comprehension in images, illustrated by the improved performance on the RefCOCO dataset. Our project is available at https://sijiesong.github.io/co-grounding.
Seeing in Extra Darkness Using a Deep-Red Flash
Jinhui Xiong KAUST, Jian Wang Snap Research, Wolfgang Heidrich KAUST, Shree Nayar Snap Research and Columbia University
Abstract
We propose a new flash technique for low-light imaging, using deep-red light as an illuminating source. Our main observation is that in a dim environment, the human eye mainly uses rods for the perception of light, which are not sensitive to wavelengths longer than 620nm, yet the camera sensor still has a spectral response. We propose a novel modulation strategy when training a modern CNN model for guided image filtering, fusing a noisy RGB frame and a flash frame. This fusion network is further extended for video reconstruction. We have built a prototype with minor hardware adjustments and tested the new flash technique on a variety of static and dynamic scenes. The experimental results demonstrate that our method produces compelling reconstructions, even in extra dim conditions.
UC2: Universal Cross-Lingual Cross-Modal Vision-and-Language Pre-Training
Mingyang Zhou University of California, Davis, Luowei Zhou Microsoft Dynamics 365 AI Research, Shuohang Wang Microsoft Dynamics 365 AI Research, Yu Cheng Microsoft Dynamics 365 AI Research, Linjie Li Microsoft Dynamics 365 AI Research, Zhou Yu University of California, Davis and Columbia University, Jingjing Liu Microsoft Dynamics 365 AI Research
Abstract
Vision-and-language pre-training has achieved impressive success in learning multimodal representations between vision and language. To generalize this success to non-English languages, we introduce UC^2, the first machine translation-augmented framework for cross-lingual cross-modal representation learning. To tackle the scarcity problem of multilingual captions for image datasets, we first augment existing English-only datasets with other languages via machine translation (MT). Then we extend the standard Masked Language Modeling and Image-Text Matching training objectives to multilingual setting, where alignment between different languages is captured through shared visual context (eg. using image as pivot). To facilitate the learning of a joint embedding space of images and all languages of interest, we further propose two novel pre-training tasks, namely Maksed Region-to-Token Modeling (MRTM) and Visual Translation Language Modeling (VTLM), leveraging MT-enhanced translated data. Evaluation on multilingual image-text retrieval and multilingual visual question answering benchmarks demonstrates that our proposed framework achieves new state of the art on diverse non-English benchmarks while maintaining comparable performance to monolingual pre-trained models on English tasks.
Learning Goals From Failure
Dave Epstein Columbia University and Carl Vondrick Columbia University
Abstract
We introduce a framework that predicts the goals behind observable human action in video. Motivated by evidence in developmental psychology, we leverage video of unintentional action to learn video representations of goals without direct supervision. Our approach models videos as contextual trajectories that represent both low-level motion and high-level action features. Experiments and visualizations show our trained model is able to predict the underlying goals in video of unintentional action. We also propose a method to “automatically correct” unintentional action by leveraging gradient signals of our model to adjust latent trajectories. Although the model is trained with minimal supervision, it is competitive with or outperforms baselines trained on large (supervised) datasets of successfully executed goals, showing that observing unintentional action is crucial to learning about goals in video.
Generative Interventions for Causal Learning
Chengzhi Mao Columbia University, Augustine Cha Columbia University, Amogh Gupta Columbia University, Hao Wang Rutgers University, Junfeng Yang Columbia University, Carl Vondrick Columbia University
Abstract
We introduce a framework for learning robust visual representations that generalize to new viewpoints, backgrounds, and scene contexts. Discriminative models often learn naturally occurring spurious correlations, which cause them to fail on images outside of the training distribution. In this paper, we show that we can steer generative models to manufacture interventions on features caused by confounding factors. Experiments, visualizations, and theoretical results show this method learns robust representations more consistent with the underlying causal relationships. Our approach improves performance on multiple datasets demanding out-of-distribution generalization, and we demonstrate state-of-the-art performance generalizing from ImageNet to ObjectNet dataset.
Learning the Predictability of the Future
Didac Suris Columbia University, Ruoshi Liu Columbia University, Carl Vondrick Columbia University
Abstract
We introduce a framework for learning from unlabeled video what is predictable in the future. Instead of committing up front to features to predict, our approach learns from data which features are predictable. Based on the observation that hyperbolic geometry naturally and compactly encodes hierarchical structure, we propose a predictive model in hyperbolic space. When the model is most confident, it will predict at a concrete level of the hierarchy, but when the model is not confident, it learns to automatically select a higher level of abstraction. Experiments on two established datasets show the key role of hierarchical representations for action prediction. Although our representation is trained with unlabeled video, visualizations show that action hierarchies emerge in the representation.
Linear Semantics in Generative Adversarial Networks
Jianjin Xu Columbia University, Changxi Zheng Columbia University
Abstract
Generative Adversarial Networks (GANs) are able to generate high-quality images, but it remains difficult to explicitly specify the semantics of synthesized images. In this work, we aim to better understand the semantic representation of GANs, and thereby enable semantic control in GAN’s generation process. Interestingly, we find that a well-trained GAN encodes image semantics in its internal feature maps in a surprisingly simple way: a linear transformation of feature maps suffices to extract the generated image semantics. To verify this simplicity, we conduct extensive experiments on various GANs and datasets; and thanks to this simplicity, we are able to learn a semantic segmentation model for a trained GAN from a small number (e.g., 8) of labeled images. Last but not least, leveraging our finding, we propose two few-shot image editing approaches, namely Semantic-Conditional Sampling and Semantic Image Editing. Given a trained GAN and as few as eight semantic annotations, the user is able to generate diverse images subject to a user-provided semantic layout, and control the synthesized image semantics. We have made the code publicly available.
Google Research held an online workshop on the conceptual understanding of deep learning. The workshop discussed how new findings in deep learning and neuroscience can help create better artificial intelligence systems. Christos Papadimitriou discussed how our growing understanding of information-processing mechanisms in the brain might help create algorithms that are more robust in understanding and engaging in conversations. Papadimitriou presented a simple and efficient model that explains how different areas of the brain inter-communicate to solve cognitive problems.
Shuran Song and Carl Vondrick are among the awardees chosen for their artificial intelligence (AI) research. The program aims to use AI for societal good.
Research from the department was accepted to the 35th AAAI Conference on Artificial Intelligence. The conference promotes research in artificial intelligence (AI) and scientific exchange among AI researchers, practitioners, scientists, and engineers in affiliated disciplines.
Automated Symbolic Law Discovery: A Computer Vision Approach
Hengrui Xing Columbia University, Ansaf Salleb-Aouissi Columbia University, Nakul Verma Columbia University
One of the most exciting applications of modern artificial intelligence is to automatically discover scientific laws from experimental data. This is not a trivial problem as it involves searching for a complex mathematical relationship over a large set of explanatory variables and operators that can be combined in an infinite number of ways. Inspired by the incredible success of deep learning in computer vision, the authors tackle this problem by adapting various successful network architectures into the symbolic law discovery pipeline. The novelty of this new approach is in (1) encoding the input data as an image with super-resolution, (2) developing an appropriate deep network pipeline, and (3) predicting the importance of each mathematical operator from the relationship image. This allowed to prior the exponentially large search with the predicted importance of the symbolic operators, which can significantly accelerate the discovery process.
The model was then applied to a variety of plausible relationships—both simulated and from physics and mathematics domains—involving different dimensions and constituents. The authors show that their model is able to identify the underlying operators from data, achieving a high accuracy and AUC (91% and 0.96 on average resp.) for systems with as many as ten independent variables. Their method significantly outperforms the current state of the art in terms of data fitting (R^2), discovery rate (recovering the true relationship), and succinctness (output formula complexity). The discovered equations can be seen as first drafts of scientific laws that can be helpful to the scientists for (1) hypothesis building, and (2) understanding the complex underlying structure of the studied phenomena. This novel approach holds a real promise to help speed up the rate of scientific discovery.
Bounding Causal Effects on Continuous Outcome
Junzhe Zhang Columbia University, Elias Bareinboim Columbia University
One of the most common methods for policy learning used throughout the empirical sciences is the use of randomization of the treatment assignment. This method is considered the gold standard within many disciplines and can be traced back, at least, to Fisher (Fisher 1935) and Neyman (Neyman 1923). Whenever human subjects are at the center of the experiment, unfortunately, issues of non-compliance arise. Namely, subjects do not necessarily follow the experimental protocol and end up doing what they want. It is well-understood that under such conditions, unobserved confounding bias will emerge. For instance, subjects who did not comply with the treatment assignment may be precisely those who would have responded adversely to the treatment. Therefore, the actual causal effects of the treatment, when it is applied uniformly to the population, might be substantially less effective than the data reveals. Moreover, since one does not observe how subjects decide/respond to the realized treatment, the actual treatment effects are not uniquely computably from the collected data, called non-identifiable.
Robins (1989) and Manski (1990) derived the first informative bounds over the causal effects from studies with imperfect compliance under a set of non-parametric assumptions called instrumental variables (IV). In their seminal work, Balke and Pearl (1994a, 1997) improved earlier results by employing an algebraic method to derive analytic expressions of the causal bounds, which are provably optimal. However, this approach assumes the primary outcome to be discrete and finite. Solving such a program could be intractable when high-dimensional context variables are present.
This paper presents novel non-parametric methods to bound causal effects on the continuous outcome from studies with imperfect compliance. These methods could be generalized to settings with a high-dimensional context. Perhaps surprisingly, this paper introduced a latent data representation that could characterize all constraints on the observational and interventional distributions implied by IV assumptions, even when the primary outcome is continuous. Such representation allows one to reduce the original bounding problem to a series of linear programs. Solve these programs, therefore, leads to tight causal bounds.
Estimating Identifiable Causal Effects through Double Machine Learning
Yonghan Jung, Jin Tian, Elias Bareinboim Columbia University
Learning causal effects from observational data is a pervasive challenge found throughout the data-intensive sciences. General methods of determining the identifiability of causal effect from a combination of observational data and causal knowledge about the underlying system have been well-understood in theory. In practice, however, there are still challenges to estimating identifiable causal functionals from finite samples. Recently, a novel approach, named double/debiased machine learning (DML) (Chernozhukov et al. 2018), has been proposed to learn parameters leveraging modern machine learning techniques, which are both robust to model misspecification (‘doubly robust’) and slow convergence (‘debiased’). Still, DML has only been used for causal estimation in settings when the back-door condition (also known as conditional ignorability) holds.
This paper aims to bridge this gap by developing a general class of estimators for any identifiable causal functionals that exhibit robustness properties of DML estimators, which the authors called ‘DML-ID.’ In particular, they provide a complete procedure for deriving an essential ingredient of the DML estimator called an influence function (IF) and construct a general class of estimators based on the IF. This means that one can estimate any causal functional and enjoy two robustness properties, doubly robustness and debiasedness.
Ref-NMS: Breaking Proposal Bottlenecks in Two-Stage Referring Expression Grounding
Long Chen Tencent AI Lab, Wenbo Ma Zhejiang University, Jun Xiao Zhejiang University, Hanwang Zhang Nanyang Technological University, Shih-Fu Chang Columbia University
The prevailing framework for solving referring expression grounding is based on a two-stage process: 1) detecting proposals with an object detector and 2) grounding the referent to one of the proposals. Existing two-stage solutions mostly focus on the grounding step, which aims to align the expressions with the proposals.
In this paper, the researchers argue that these methods overlook an obvious mismatch between the roles of proposals in the two stages: they generate proposals solely based on the detection confidence (i.e., expression-agnostic), hoping that the proposals contain all right instances in the expression (i.e., expression-aware). Due to this mismatch, current two-stage methods suffer from a severe performance drop between detected and ground-truth proposals.
The paper proposes Ref-NMS, which is the first method to yield expression-aware proposals at the first stage. Ref-NMS regards all nouns in the expression as critical objects, and introduces a lightweight module to predict a score for aligning each box with a critical object. These scores can guide the NMS operation to filter out the boxes irrelevant to the expression, increasing the recall of critical objects, resulting in a significantly improved grounding performance.
Since RefNMS is agnostic to the grounding step, it can be easily integrated into any state-of-the-art two-stage method. Extensive ablation studies on several backbones, benchmarks, and tasks consistently demonstrate the superiority of Ref-NMS. Codes are available at: https://github.com/ChopinSharp/ref-nms.
Professor Kathy McKeown talks with DeepLearning.AI’s Andrew Ng about how she started in artificial intelligence (AI), her research projects, how her understanding of AI has changed through the decades, and AI career advice for learners of NLP.